AI can reliably spot molecules on exoplanets, and might one day even discover new laws of physics (Image Credit: SNN)
Do you know what the Earth’s atmosphere is made of? You’d probably remember it’s oxygen, and maybe nitrogen. And with a little help from Google you can easily reach a more precise answer: 78% nitrogen, 21% oxygen and 1% Argon gas. However, when it comes to the composition of exo-atmospheres—the atmospheres of planets outside our solar system—the answer is not known. This is a shame, as atmospheres can indicate the nature of planets, and whether they can host life.
As exoplanets are so far away, it has proven extremely difficult to probe their atmospheres. Research suggests that artificial intelligence (AI) may be our best bet to explore them—but only if we can show that these algorithms think in reliable, scientific ways, rather than cheating the system. Now our new paper, published in The Astrophysical Journal, has provided reassuring insight into their mysterious logic.
Astronomers typically exploit the transit method to investigate exoplanets, which involves measuring dips in light from a star as a planet passes in front of it. If an atmosphere is present on the planet, it can absorb a very tiny bit of light, too. By observing this event at different wavelengths—colors of light—the fingerprints of molecules can be seen in the absorbed starlight, forming recognizable patterns in what we call a spectrum. A typical signal produced by the atmosphere of a Jupiter-sized planet only reduces the stellar light by ~0.01% if the star is Sun-like. Earth-sized planets produce 10–100 times lower signals. It’s a bit like spotting the eye color of a cat from an aircraft.
In the future, the James Webb Space Telescope (JWST) and the Ariel Space Mission, both probes that will investigate exoplanets from their orbit in space, will help by providing high-quality spectra for thousands of exo-atmospheres. But while scientists are excited about this, the latest research suggests it may be tricky. Due to the complex nature of atmospheres, the analysis of a single transiting planet may take days or even weeks to complete.
Naturally, researchers have started to look for alternative tools. AI are renowned for their ability to assimilate and learn from a large amount of data and their superb performance on different tasks once trained. Scientists have therefore attempted to train AI to predict the abundance of various chemical species in atmospheres.
Current research has established that AIs are well-suited for this task. However, scientists are meticulous and skeptical, and to prove this is really the case, they want to understand how AIs think.
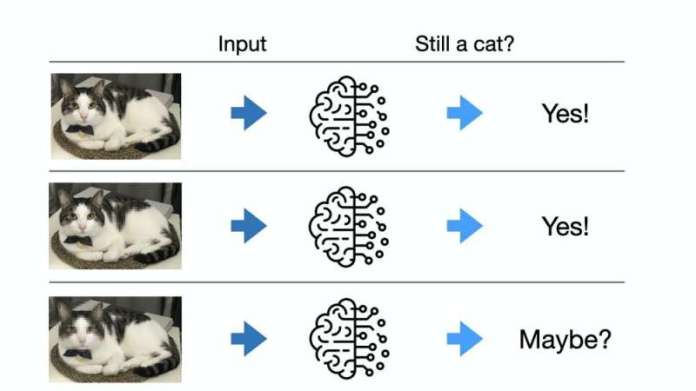
Peeking inside the black box
In science, a theory or a tool cannot be adopted if it is not understood. After all, you don’t want to go through the excitement of discovering life on an exoplanet, just to realize it is simply a “glitch” in the AI. The bad news is that AIs are terrible at explaining their own findings. Even AI experts have a hard time identifying what causes the network to provide a given explanation. This disadvantage has often prevented the adoption of AI techniques in astronomy and other scientific fields.
We developed a method that allows us a glimpse into the decision-making process of AI. The approach is quite intuitive. Suppose an AI has to confirm whether an image contains a cat. It would presumably do this by spotting certain characteristics, such as fur or face shape. To understand which characteristics it is referencing, and in what order, we could blur parts of the cat’s image and see if it still spots that it is a cat.
This is exactly what we did for an exoplanet-probing AI by “perturbing,” or changing, regions of the spectrum. By observing how the AI’s predictions on the abundances of exoplanet molecules changed (say water in the atmosphere) when each region was doctored, we started to build a “picture” of how the AI thought, such as which regions of the spectrum it used for deciding the level of water in the atmosphere.
Reassuringly for us astronomers, we found that a well-trained AI relies heavily on physical phenomena, such as unique spectroscopic fingerprints—just like an astronomer would. This may come as no surprise, after all, where else can the AI learn it from? In fact, when it comes to learning, AI is not so different from a cheeky high-school student—it will try its best to avoid the hard way (such as understanding difficult mathematical concepts) and find any shortcuts (such as memorizing the mathematical formulae without understanding why) in order to get the correct answer. If the AI made predictions based on memorizing every single spectrum it had come across, that would deeply undesirable. We want the AI to derive its answer from the data, and perform well on unknown data, not just the training data for which there is a correct answer.
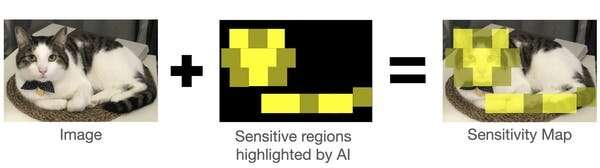
This finding provided the first method to have a sneaky peek into so-called “AI black-boxes,” allowing us to evaluate what the AIs have learnt. With these tools, researchers now can not only use AIs to speed up their analysis of exo-atmospheres, but they can also verify that their AI uses well-understood laws of nature.
That said, it’s too early to claim that we fully understand AIs. The next step is to work out precisely how important each concept is, and how it gets processed into decisions.
The prospect is exciting for AI experts, but even more so for us scientists. AI’s incredible learning power originates from its ability to learn a “representation,” or pattern, from the data—a technique similar to how physicists have discovered laws of nature in order to better understand our world. Having access to the minds of AI may therefore grant us the opportunity to learn new, undiscovered laws of physics.
– Advertisement –