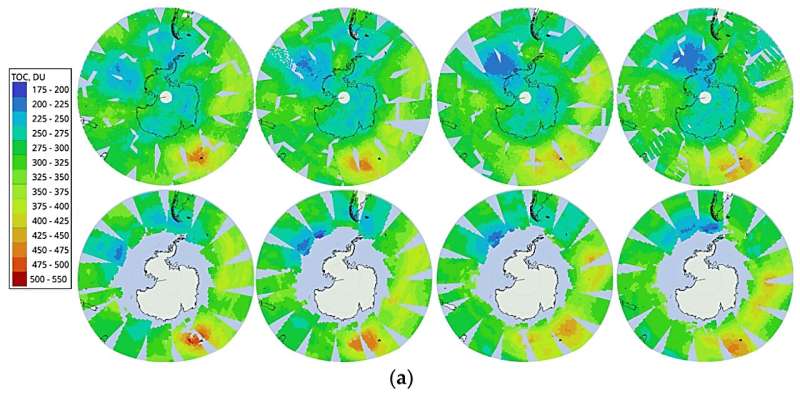
A team of scientists from St Petersburg University, in collaboration with their colleagues from the Scientific-Research Centre “Planeta” and the Keldysh Research Centre, has developed a neural network algorithm for measuring the ozone content in the atmosphere, using data from a Russian meteorological satellite.
The study was carried out in the Ozone Layer and Upper Atmosphere Research Laboratory at St Petersburg University.
Ozone is a gaseous substance that is found in the Earth’s atmosphere and is mainly concentrated at altitudes between 10 and 50 kilometers. Ozone plays a vital role as it absorbs ultraviolet radiation from the sun and protects living organisms on the Earth’s surface from the harmful effects of this radiation. Additionally, it influences chemical reactions in the upper layers of the Earth’s atmosphere and helps regulate the Earth’s temperature by absorbing some of the sun’s heat.
The IKFS-2 spectrometer for meteorological atmospheric sounding, installed on the Russian Meteor M series satellites, measures the spectra of outgoing radiation, which contain not only meteorological information but also data on the composition of the atmosphere.
Alexander Polyakov is Professor at St Petersburg University and Research Associate in the Ozone Layer and Upper Atmosphere Research Laboratory at the University. According to him, the IKFS-2 spectrometer has been working aboard the Meteor M series of meteorological satellites for eight years and has not lost its relevance. The scientists from St Petersburg University have developed a technology that can be applied to this series of satellites.
The algorithm and code developed by our research team are directly applicable to the instruments on board the next Meteor M series satellites, the next of which is scheduled for launch this summer. The algorithm can also be adapted to similar instruments on board other weather satellites.
Alexander Polyakov, Professor at St Petersburg University and Research Associate in the Ozone Layer and Upper Atmosphere Research Laboratory at St Petersburg University
The neural network underlying the retrieval algorithm developed by the researchers from St Petersburg University was trained on data obtained over six years of observations. More than 19,000,000 spectra measured by the IKFS-2 instrument, combined with measurements of total ozone columns in the atmosphere from the Aura satellite, were processed for this purpose.
The results of ozone measurements obtained using the algorithm developed by the physicists for the IKFS-2 spectral measurements were compared with data from ground-based instruments and satellites specially designed for measurements of total ozone columns: the differences do not exceed 3%.
For reference: earlier, the researchers from the Ozone Layer and Upper Atmosphere Research Laboratory of St Petersburg University found that magnetic storms destroy up to a quarter of the earth’s ozone layer in the mesosphere in one day. The recorded destruction is at an altitude of about 75 km.
More information:
Alexander Polyakov et al, Six Years of IKFS-2 Global Ozone Total Column Measurements, Remote Sensing (2023). DOI: 10.3390/rs15092481
Provided by
St. Petersburg State University
Physicists develop a technology for measuring total ozone columns based on machine learning (2023, August 2)
retrieved 27 September 2023
from https://phys.org/news/2023-08-physicists-technology-total-ozone-columns.html
part may be reproduced without the written permission. The content is provided for information purposes only.