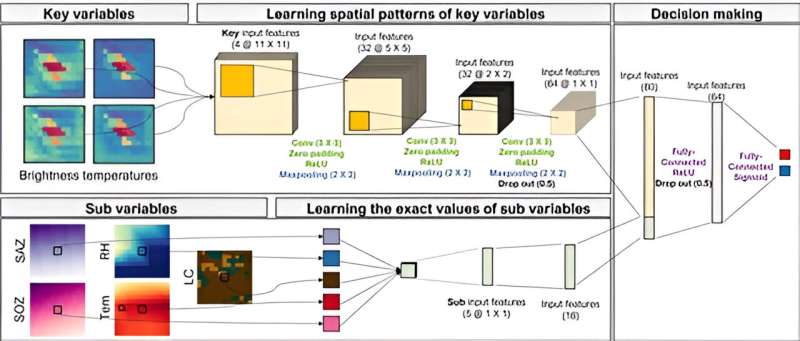
A technology that combines satellite data and numerical model data for forest fire detection has been developed, offering a more comprehensive and adaptable approach to monitor and respond to wildfires. This innovative solution, developed by Professor Jungho Im and his team in the Department of Civil, Urban, Earth, and Environmental Engineering at UNIST, has the potential to significantly minimize the damage caused by medium and large forest fires.
Traditional wildfire detection systems have relied solely on satellite data for over two decades. However, the research team led by Professor Im sought to enhance the existing approach by integrating numerical model data used in weather forecasting. By combining various data, including relative humidity, surface temperature, and satellite observation angle, the team developed a deep learning model with a dual-module convolutional neural network (DM CNN) structure to independently extract and combine satellite and numerical model data.
The developed technology was compared to widely used detection technologies such as MODIS/VIIRS, AHI, and AMI. Existing methods struggle to accurately detect forest fires due to mixed signals caused by factors like humidity and sun position. In contrast, the model developed by Professor Im’s team considers multiple variables simultaneously, providing a significant advantage in maintaining detection accuracy despite changes in the environment.
Real-world experiments were conducted to validate the technology’s performance under various environmental conditions. The results demonstrated that the developed model outperformed existing detection methods, showcasing its ability to more accurately locate wildfires. Although the satellite resolution is lower compared to narrow-range detection technologies, the wider spatial range covered by the model (4㎢) compensates for this by offering higher accuracy.
“This study maximizes the advantages of deep learning, enabling the convergence of heterogeneous data with diverse characteristics,” stated Professor Im. “It represents a significant achievement in proposing a new direction for global forest fire detection technology.”
The work is published in the journal Remote Sensing of Environment.
More information:
Yoojin Kang et al, Toward an adaptable deep-learning model for satellite-based wildfire monitoring with consideration of environmental conditions, Remote Sensing of Environment (2023). DOI: 10.1016/j.rse.2023.113814
Provided by
National Institute of Standards and Technology
New study unveils breakthrough in forest fire detection despite environmental changes (2023, October 20)
retrieved 21 October 2023
from https://phys.org/news/2023-10-unveils-breakthrough-forest-environmental.html
part may be reproduced without the written permission. The content is provided for information purposes only.